Are you fascinated by the recent hype around data and artificial intelligence (AI)? Apart from leveraging external tools like Chat GPT, are you wondering how to implement your own machine learning (ML) projects in your organization? And how do you take projects from the PoC and experimentation stage into production, including deploying and continuously running, monitoring, and improving your ML systems?
Artificial intelligence (AI) and machine learning (ML) are key drivers of digital transformations in today's organizations. Many companies want to benefit from AI and ML in hopes of turning data into value. Many models and projects fail, however, to yield reliable results when deployed. Others start well, but results erode, leaving their owners, collaborators, and users frustrated. Many businesses do not get the return on investment they expect.
Two critical aspects are continuous data quality management and MLOps (machine learning development and operations), but the question remains: How can you successfully implement an end-to-end approach for reliable and continuous machine learning delivery and operations?
In this webinar, we present our blueprint for a digital highway for ML systems to address that question and illustrate how organizations can generate sustainable value by building and running reliable machine learning solutions.
What will be covered
- Why are reliability, scalability, cross-functional collaboration, and an end-to-end approach, including deployment, service level management, continuous quality assurance, and learning from failure, crucial for ML projects?
- How can you design and build a digital highway for ML systems, including end-to-end pipelines for data, model, and code to implement continuous delivery for machine learning?
- What are best practices for running business-critical “traditional” software that we can leverage for ML systems? And what is different about ML systems?
- ... and how can you assess where your team and organization stand today, and what steps to take next are relevant to you?
Pre-requisites
Interest in and understanding of data and machine learning-related challenges and opportunities. We will explain a few technical concepts, but no technical knowledge is required. This will be an educational introduction webinar.
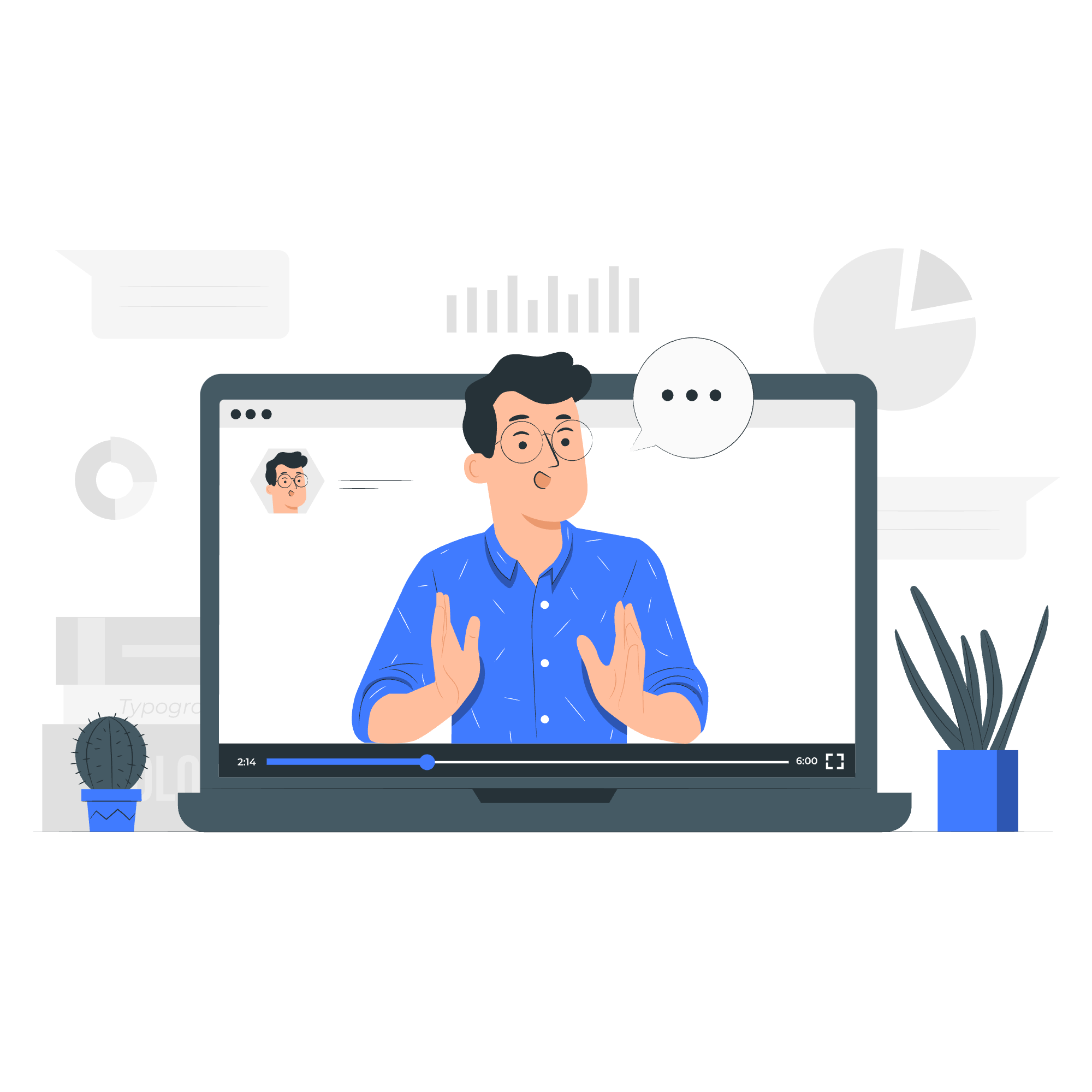
Our Speakers
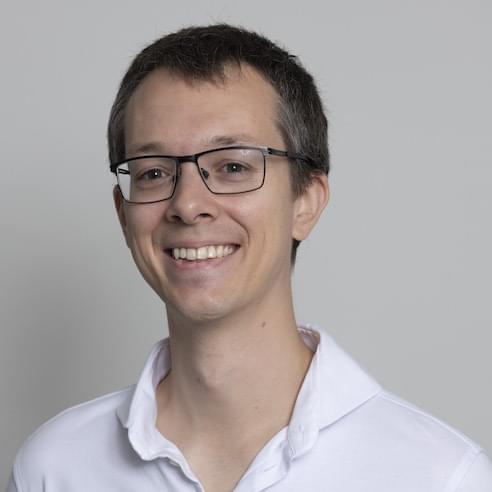
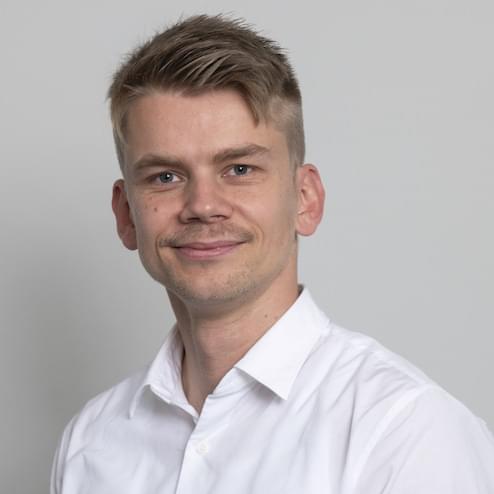
Join Our Webinar
This webinar is part of our series of blog posts and webinars called
Next Generation Data & AI Journey powered by MLOps
Learn more about our expertise here and sign up for our newsletter.
Join Us