The use of Machine Learning (ML) and its operationalization through the Machine Learning Operations (MLOps) paradigm bring a lot of benefits. Business decisions can increasingly be made based on or aided by data and automated methods, inferring information from that data. Tedious and repetitive tasks can be completed without human interaction or new business cases can be implemented more efficiently. While Machine Learning solves the algorithmic problem of a use case, MLOps concerns itself with operationalizing such an algorithm (e.g., serving it through an API infrastructure, continuously improving the algorithm, managing the data for the algorithm, etc.). For clarity, we call embedding the algorithm in a MLOps framework a “production-level Machine Learning application”.
However, to reach production, a business must overcome a vast number of challenges to successfully leverage the potential of machine learning. In our last blog post (see Effective MLOps in Action), we have presented a concrete implementation of an MLOps use case, predicting breast cancer from patient data. This implementation covers the technical aspects in depth; however, it does not consider other aspects of an end-to-end lifecycle within a real-life scenario such as planning, operating, and improving such a solution to optimize for business value.
Effective MLOps Scope
From an engineer's point of view, the main tasks to develop a production-ready ML application are primarily limited to data modeling and prediction generation. From the perspective of the business that runs and relies on an ML system, the challenges are more intricate. Consider the following questions:
- Do we have the resources to sustainably make use of the new system?
- How will the changes be perceived by our employees and/or customers?
- How can such a method be operationalized in the day-to-day business?
- How can we guarantee sustainable prediction quality?
- How can we achieve long-term improvements of the new system?
- How can we approach cross-functional challenges arising from the new system (e.g., challenges that require technical and business knowledge to be solved)?
- How can the business value be maximized from a technical implementation of a production-level Machine Learning application?
Essentially, all these challenges fall into three different dimensions: Technologies, Culture & Skills, and Operating Model. Considering all three aspects when planning and implementing an ML solution, allows for a holistic reflection on the challenges a business might face.
Holistic considerations for a production-ready ML application
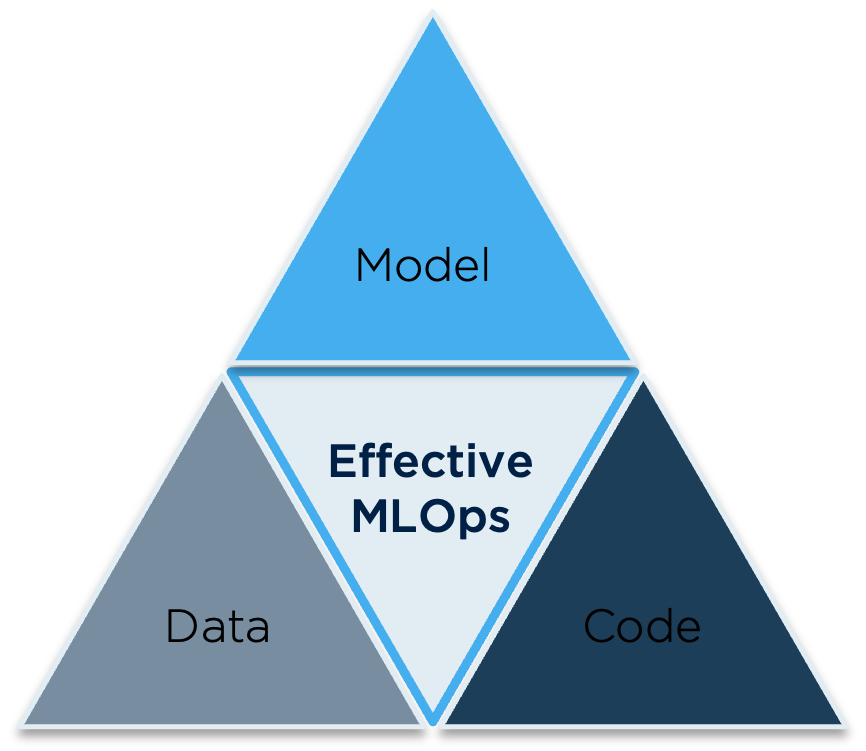
Different stakeholders have different interests and prerequisites concerning technological advances within a business. While a shareholder aims for the general improvement of efficiency, an office employee would like to spend as little time on monotonous tasks as possible. The head of sales, on the other hand, concerns himself with obtaining deeper insights into the company's customer base to be able to target them with more relevant products.
This diversity of interests underlines the importance of involving all stakeholders when implementing the changes accompanying the introduction of an ML solution (rather than in a top-down fashion). This ensures that improvements are as expedient as possible, but also requires a business to approach challenges in digitalization in a holistic way. In the case of a production-ready Machine Learning application, the relevant aspects for a holistic analysis correspond to the three “Effective MLOps” dimensions.
- Data: The dimension of Data focuses on managing the data lifecycle, ensuring high-quality data, and enabling smooth data integration and processing for ML models.
- Model: The dimension of Model focuses on managing ML models throughout their lifecycle, including training, evaluation, monitoring, optimization, and explainability.
- Code: The dimension of Code focuses on integrating data-, and ML-based solutions into the software development and deployment lifecycle, enabling seamless collaboration and continuous delivery.
As a business, it is key to understand, where improvements must be made to assure that the aforementioned points are satisfied. Also, understanding the maturity of each aspect of the MLOps scope allows for better decision-making when it comes to determining feasibility and requirements for production-level Machine Learning projects. Additionally, it enables measuring progress with solid success criteria. So how can maturity be measured holistically?
Theoretical Frameworks for Maturity
Maturity with respect to MLOps can be measured in an abundance of ways. Google has published its own model for MLOps maturity in 2020. This model presents three different maturity levels. The first maturity (Level 0) can be described as applying each step in the pipeline, from data analysis to serving the model, manually. The second maturity (Level 1) facilitates continuous training as the model is automatically re-trained in production. Other than Level 0, Level 1 deploys a whole training pipeline instead of just a trained model with a corresponding service to production. It is sufficient if data changes often, but the ML approach does not. Level 2 focuses on improving continuous integration and continuous delivery of the pipeline. This level of maturity is a requirement for a functional production-level Machine Learning application, when not only the data changes frequently, but also the ML model.
Deloitte clams to have created the first pan-organizational digital maturity model in 2018, which considers the five core dimensions “Customer”, “Strategy”, “Technology”, “Operations” and “Organization & Culture”. Their maturity model follows a holistic approach to digitalization and innovation, but it does not specifically consider MLOps.
Microsoft presented its own maturity model for MLOps in 2020. They present five distinct levels (“Level 0: No MLOps”, “Level 1: DevOps but no MLOps”, “Level 2: Automated Training”, “Level 3: Automated Model Deployment” and “Level 4: Full MLOps Automated Operations”). Yet again, that model only focuses solely on the technological aspect of the three “Effective MLOps Scopes”.
Before we present our own maturity model, it is essential to understand what the three dimensions, “Data”, “Model” and “Code”, entail:
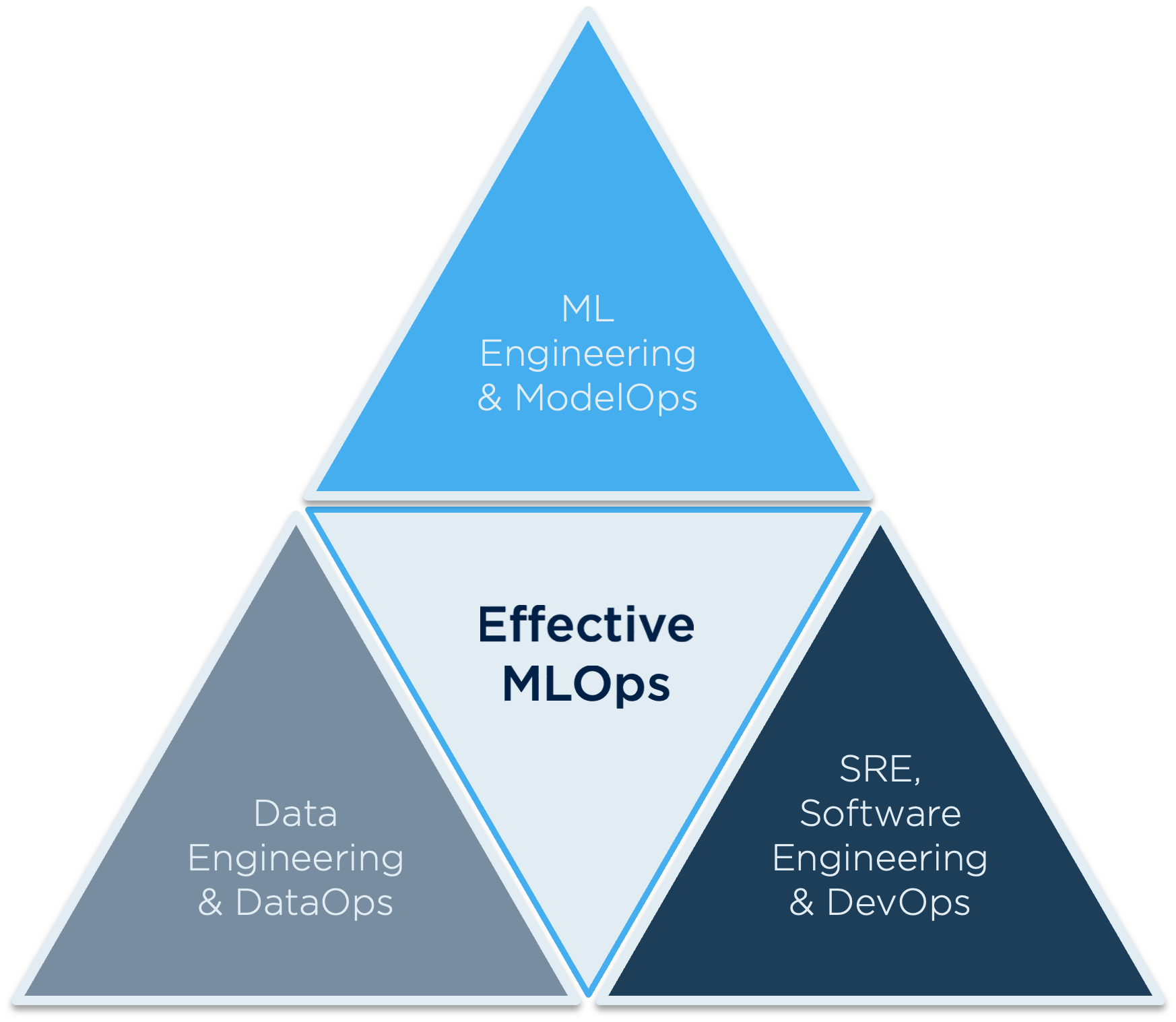
- Data Engineering & DataOps: are integral to the end-to-end ML lifecycle, covering data collection, storage, transformation, governance, and analytics. These disciplines ensure that ML workflows are supported by efficient and robust data pipelines, enabling organizations to leverage high-quality data for successful ML model development and delivery.
- ML Engineering & ModelOps: play critical roles in the end-to-end ML lifecycle, focusing on the development, deployment, and management of machine learning models. By incorporating ML Engineering and ModelOps practices, organizations can effectively develop, deploy, and manage machine learning models throughout their lifecycle. These disciplines ensure that models are developed and validated with high quality, deployed with reliability, monitored for performance, and updated as needed to deliver accurate predictions in production environments.
- Software Engineering & DevOps: refer to the ability of continuously improve, automate and establish well-defined processes with respect to data, code and model. In this context of an end-to-end ML lifecycle, this could be exemplified by how well a company applies the principles of DataOps (data versioning) and ModelOps (model and experiment tracking and continuous training). These principles contribute to efficient software development, reliable infrastructure management, continuous integration, monitoring, and delivery, resulting in robust and scalable ML solutions.
Data, AI & ML (Ops) Transformation Journey
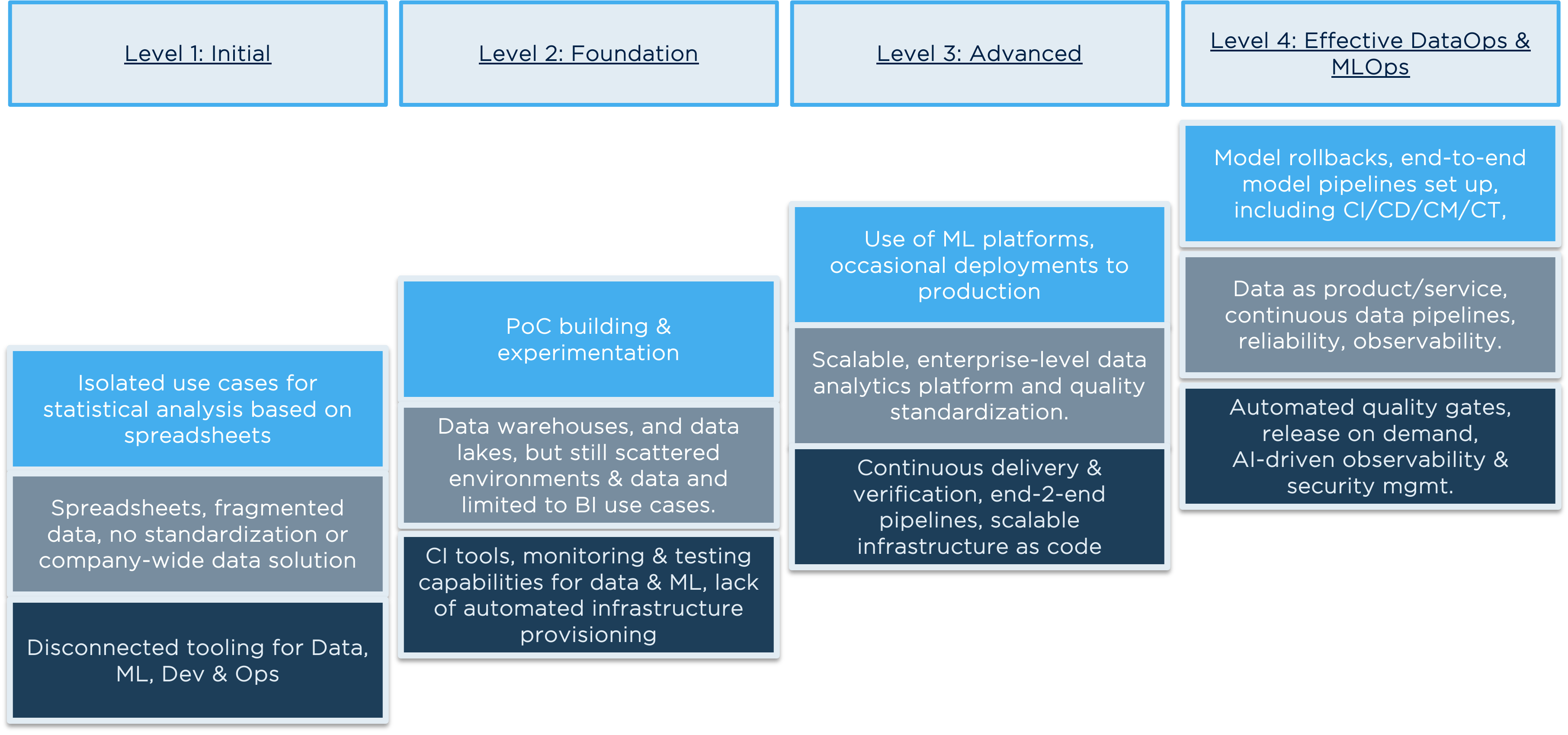
Our model consists of three dimensions presented in the sections above: model (light blue), data (grey), and code (dark blue). For each of these dimensions we present the progression of capabilities and best practices for organizations to assess and improve their MLOps practices. It provides a roadmap for organizations to understand their current state, identify areas of improvement, and define a path towards achieving maturity in their MLOps implementation. The model typically consists of different levels, as presented in Figure 3, each representing increasing levels of maturity and sophistication. Their meaning is as follows:
Level 1
Model: Isolated use cases for statistical analysis based on spreadsheets at Level 1 of the MLOps maturity model typically involve manual data entry and analysis using basic spreadsheet software. These use cases lack standardized processes, version control, and automation, resulting in limited scalability. The analysis is often performed in silos without integration into a larger data pipeline or cohesive ML workflow.
Data: The most rudimentary way to manage data is to store it locally, either on a computer or on a company-hosted local server. This can lead to difficulties in versioning files, access, data loss, etc. At level 1, data is stored on a data server, which partially addresses the problems of data accessibility and file versioning. Nevertheless, it still exposes the company to the possibility of data loss since the data is not redundantly stored. In the early stages of maturity, a significant issue arises where data becomes fragmented. Similar data is stored in multiple locations due to a lack of process standardization. Although the data could potentially be stored on the same server or file system, it is distributed across different locations within that server.
Code: At level 1 the problem is the use of separate and disparate tools for various aspects of the data lifecycle, machine learning, development, and operations processes. In this scenario, different teams or individuals may use different tools that are not well-integrated or compatible with each other, resulting in fragmentation, inefficient workflows, as well as a limited ability to streamline and scale operations.
Level 2
Model: At this maturity level, an organization has a systematic approach to building proof of concepts (PoCs) and conducting experimentation to explore new solutions and technologies. There is a structured process for model development and testing, allowing the organization to validate hypotheses, assess feasibility, and implement ML-based solution that can be delivered in a production environment.
Data: At level 2, a company makes use of cloud infrastructure to store data, as well as high-performance databases, allowing for lower latency when accessing data for training. This indicates an intermediate stage in the data management journey. Despite the presence of data warehouses and data lakes, the overall data environment remains scattered. Data may be spread across different systems, databases, and storage platforms, making it challenging to have a unified view of the data and inhibiting efficient data integration and analysis.
Code: An organization at this stage has implemented continuous integration (CI) tools and practices to automate the data and model pipelines. In addition, monitoring and testing is applied at this level to track the performance and quality of data and model processes. However, the infrastructure provisioning still requires manual intervention and lacks of automation and scalability.
Level 3
Model: At level 3, an organization has adopted ML platforms that provide infrastructure and tools for developing, training, and deploying machine learning models. However, there is still space for improvements in terms of scalability, automation, and integration of ML models into the core business processes, despite the occasional deployments in production.
Data: At this maturity level, an organization is using a scalable data platform capable of handling large volumes of data and supporting complex analytical processes. It has also implemented many standardized processes for data quality management, data governance, and security, that enables analysts, and business stakeholders to gain accurate insights from the data and to drive informed decision-making at an enterprise level.
Code: At level 3, an organization has achieved a high level of automation and efficiency in delivering and verifying data and ML solutions, with automated deployment pipelines that ensure consistent and reliable releases. Although infrastructure is managed as code to enhance scalability and automations, there is still a need for leveraging AI-driven techniques to achieve a more proactive incident management, automatic remediation, and enhanced overall performance.
Level 4
Model: At this maturity level, an organization has adopted all the advanced concepts of Site Reliability Engineering and MLOps best practices. Hence, it has established robust processes for model rollbacks, in case of performance degradation, as well as end-to-end model pipelines, encompassing continuous integration (CI), continuous deployment (CD), continuous monitoring (CM), and configuration training (CT), ensuring streamlined and automated workflows for model development, deployment, monitoring, and maintenance.
Data: At this level of maturity, an organization treats data as a product recognizing the importance of driving business outcomes and bringing value to the real world. Continuous data pipelines are established to ensure an automated flow of data from various sources, enabling real-time analysis. Reliability and observability practices are implemented to monitor and ensure the quality, availability, and performance of data pipelines.
Code: At level 4, an organization has reached already a fully automated end-to-end data and model development and delivery with all required quality gates, data and ML-driven products that are scalable, reliable, and secure (see also our previous blog post about Testing & Quality Assurance (QA) for Data, ML Model and Code Pipelines). The organization has achieved the capability to release data and ML solutions on demand, with streamlined and automated processes which leverage from AI-driven observability and security management to continuously manage the stability, reliability, and security of data and ML systems. Hence, it is enhanced a proactive identification of issues, an automatic incident management, and an improved overall performance.
Advantages of assessing MLOps maturity
Our MLOps maturity model sheds light on the various aspects (both technological and non-technological) that should be considered when running MLOps at the production level. Why it is important to understand the MLOps maturity of an organization? Falling far behind in one aspect of MLOps leads to inefficiencies. Having the market-leading unified data platform, but no employee who can operate it signifies a loss of potential. The functionality of that platform will not be used to its fullest potential. Similarly, having employees capable of advanced Machine Learning while still taking reactive decisions is a waste of talent. Predictive methods could have been implemented to facilitate taking business decisions in a more reactive way. The bottom line is, that awareness about the capabilities and requirements of MLOps in a holistic way leads to improvement for any business that aims to digitize. Not only does the improved understanding facilitate planning and implementing new solutions, but also allows for concise evaluation of inefficiencies within the scope of MLOps.
Machine Learning Architects Basel
A maturity level assessment is essential for the end-to-end data and ML lifecycle to foster the organization's overall capability and readiness in effectively implementing data-, and ML-driven solutions. It helps identify gaps and areas of improvement across key dimensions such as data management, model development, deployment, monitoring, and governance. This maturity model assessment enables organizations to establish a baseline, set goals, and prioritize investments to enhance their ML capabilities and ensure successful and sustainable ML deployments.
Machine Learning Architects Basel (MLAB) is a member of the Swiss Digital Network (SDN). Having pioneered the Digital Highway for End-to-End Machine Learning & Effective MLOps we have created frameworks and reference models that combine our expertise in DataOps, Machine Learning, MLOps, and our extensive knowledge and experience in DevOps, SRE, and agile transformations.
If you want to learn more about how MLAB can aid your organization in creating long-lasting benefits by developing and maintaining reliable data and machine learning solutions, don't hesitate to contact us.
We hope you find this blog post informative and engaging. It is part of our Next Generation Data & AI Journey powered by MLOps.
References and Acknowledgements
- MLOps: Continuous delivery and automation pipelines in machine learning, by Google in 2020.
- Effective MLOps Scope, by Dr. Houssem Ben Mahfoudh in 2021.
- Effective MLOps in Action, by Dr. Houssem Ben Mahfoudh in 2021.
- Digital Maturity Model, by Deloitte in 2018.
- Machine Learning operations maturity model, by Microsoft in 2020.